The concept of eHelp is actually quite simple - the vast majority of technical support questions have already been answered so by indexing as much technical support information as possible we are in the best possible position to automatically answer your question. Whilst the general concept is not difficult, in reality the technical challenges of retrieving, processing and analysing this vast amount of data is more complex.
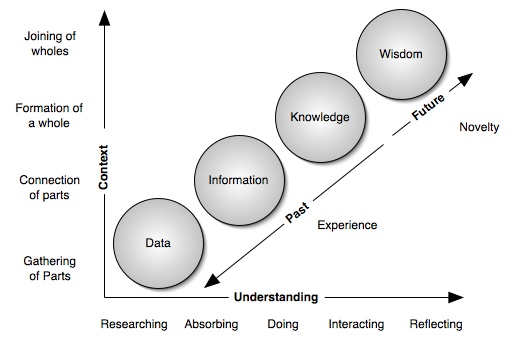
The process by which we gather, analyse and present the huge amount of technical content that we do is best described by the Data, Information, Knowledge and Wisdom approach. We gather data which might be HTML, plain text or even in binary form. Through our proprietary analysis systems we then process this; turning it into a commonly understandable form and move the data into the information realm by cross analysis. It must be said that we are selective on the data pulled forward and much of it is left 'on the cutting room floor!' Not only using our analysis suite but also through our searching technology Helpforce appears to users as knowledgeable by presenting this information to solve specific questions. We also believe in the importance of community driven contributions which help improve the quality of our knowledge. Not only by providing a technical support question answering mechanism but also allowing for the discovery of this knowledge (via eDiscover) we aim to provide our users with wisdom which equates to improved technical knowledge and the ability to make informed future decisions.
A case study
Imagine for a moment that there is an existing technical support forum, answering questions about a commonly used game; let's call this forum the ACME game support forum. When the discover aspect of Helpforce realises that there is technical information that people might find useful, then this forum is crawled for a snapshot of the HTML and text that make up the site contents. Once retrieved this data is further analysed to extract out technical support specific aspects and the rest is discarded. The next stage is to move from data and into information, this is done using a wide variety of approaches but here we will consider one specific case - poster analysis. As an example, often technical support data is posted by a specific person and if we can identify that individual (e.g. via their username) then a picture of them and their technical ability can be built up. Numerous people agreeing with them might indicate that they are to be trusted and likewise people refuting their contributions might suggest we ignore them. Even this simple technique has hidden complexity - for instance how can we match usernames across our entire data set (usernames are rarely unique) and how can this technique be applied to the entire data set in a fast and efficient manner? Once the analysis phase has been completed then the information is added into the Refined Information Store (RIS) which will be indexed by the searching system in time. Associated with all technical support content is meta data so that we can, amongst other things, correctly attribute the source of our technical support resources.
Work in progress
Whilst we have come a long way since 1998, there is still much to be done and many technical challenges still remain unsolved in this area. We are continually working to improve our coverage and the quality of our technical support. If there are certain omissions or inconsistencies then please bear in mind that we are constantly improving our techniques and due to the massive amount of data out there we certainly have our work cut out!